Catalogue Search | MBRL
نتائج البحث
MBRLSearchResults
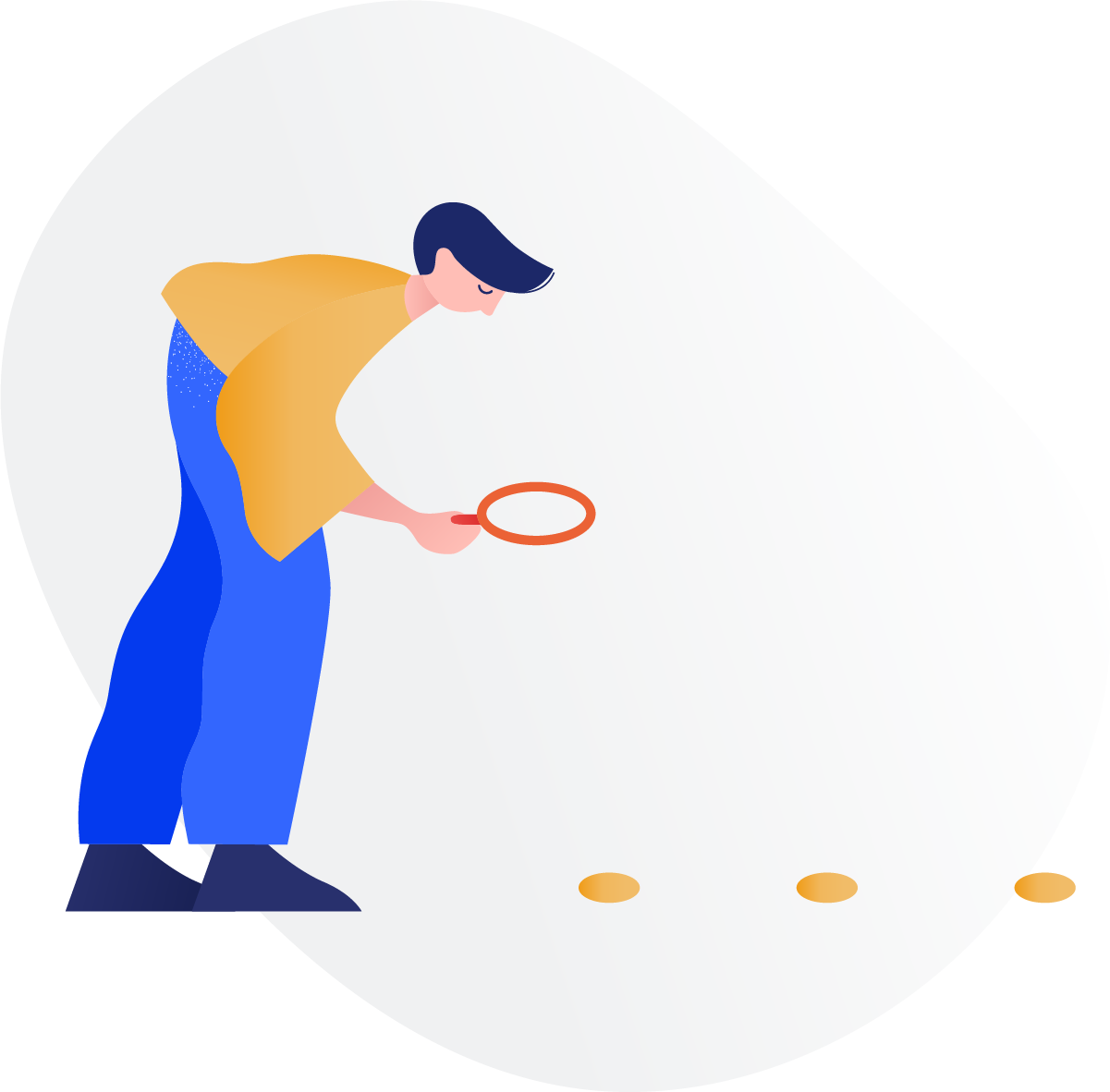
وجه الفتاة! هناك خطأ ما.
أثناء محاولة إضافة العنوان إلى الرف ، حدث خطأ ما :( يرجى إعادة المحاولة لاحقًا!
-
الضبطالضبط
-
مُحَكَّمةمُحَكَّمة
-
مستوى القراءةمستوى القراءة
-
نوع المحتوىنوع المحتوى
-
السنةمن:-إلى:
-
المزيد من المرشحاتالمزيد من المرشحاتنوع العنصرلديه النص الكاملالموضوعالناشرالمصدرالمُهدياللغةمكان النشرالمؤلفينالموقع
منجز
مرشحات
إعادة تعيين
33
نتائج ل
"Iba, Hitoshi"
صنف حسب:
Evolving robust gene regulatory networks
بواسطة
Moscato, Pablo
,
Noman, Nasimul
,
Iba, Hitoshi
في
Algorithms
,
Analysis
,
Architectural engineering
2015
Design and implementation of robust network modules is essential for construction of complex biological systems through hierarchical assembly of 'parts' and 'devices'. The robustness of gene regulatory networks (GRNs) is ascribed chiefly to the underlying topology. The automatic designing capability of GRN topology that can exhibit robust behavior can dramatically change the current practice in synthetic biology. A recent study shows that Darwinian evolution can gradually develop higher topological robustness. Subsequently, this work presents an evolutionary algorithm that simulates natural evolution in silico, for identifying network topologies that are robust to perturbations. We present a Monte Carlo based method for quantifying topological robustness and designed a fitness approximation approach for efficient calculation of topological robustness which is computationally very intensive. The proposed framework was verified using two classic GRN behaviors: oscillation and bistability, although the framework is generalized for evolving other types of responses. The algorithm identified robust GRN architectures which were verified using different analysis and comparison. Analysis of the results also shed light on the relationship among robustness, cooperativity and complexity. This study also shows that nature has already evolved very robust architectures for its crucial systems; hence simulation of this natural process can be very valuable for designing robust biological systems.
Journal Article
Evolutionary Computation in Gene Regulatory Network Research
بواسطة
Hitoshi Iba, Nasimul Noman
في
Evolutionary computation
,
Gene regulatory networks
,
Genetic algorithms
2016
Introducing a handbook for gene regulatory network research using evolutionary computation, with applications for computer scientists, computational and system biologists This book is a step-by-step guideline for research in gene regulatory networks (GRN) using evolutionary computation (EC). The book is organized into four parts that deliver materials in a way equally attractive for a reader with training in computation or biology. Each of these sections, authored by well- known researchers and experienced practitioners, provides the relevant materials for the interested readers. The first part of this book contains an introductory background to the field. The second part presents the EC approaches for analysis and reconstruction of GRN from gene expression data. The third part of this book covers the contemporary advancements in the automatic construction of gene regulatory and reaction networks and gives direction and guidelines for future research. Finally, the last part of this book focuses on applications of GRNs with EC in other fields, such as design, engineering and robotics. • Provides a reference for current and future research in gene regulatory networks (GRN) using evolutionary computation (EC) • Covers sub-domains of GRN research using EC, such as expression profile analysis, reverse engineering, GRN evolution, applications • Contains useful contents for courses in gene regulatory networks, systems biology, computational biology, and synthetic biology • Delivers state-of-the-art research in genetic algorithms, genetic programming, and swarm intelligence Evolutionary Computation in Gene Regulatory Network Research is a reference for researchers and professionals in computer science, systems biology, and bioinformatics, as well as upper undergraduate, graduate, and postgraduate students. Hitoshi Iba is a Professor in the Department of Information and Communication Engineering, Graduate School of Information Science and Technology, at the University of Tokyo, Toyko, Japan. He is an Associate Editor of the IEEE Transactions on Evolutionary Computation and the journal of Genetic Programming and Evolvable Machines. Nasimul Noman is a lecturer in the School of Electrical Engineering and Computer Science at the University of Newcastle, NSW, Australia. From 2002 to 2012 he was a faculty member at the University of Dhaka, Bangladesh. Noman is an Editor of the BioMed Research International journal. His research interests include computational biology, synthetic biology, and bioinformatics.
eBook
Pulse Detecting Genetic Circuit - A New Design Approach
بواسطة
Noman, Nasimul
,
Iba, Hitoshi
,
Inniss, Mara
في
Bacteriophage lambda - physiology
,
Biology and Life Sciences
,
Biosensing Techniques
2016
A robust cellular counter could enable synthetic biologists to design complex circuits with diverse behaviors. The existing synthetic-biological counters, responsive to the beginning of the pulse, are sensitive to the pulse duration. Here we present a pulse detecting circuit that responds only at the falling edge of a pulse-analogous to negative edge triggered electric circuits. As biological events do not follow precise timing, use of such a pulse detector would enable the design of robust asynchronous counters which can count the completion of events. This transcription-based pulse detecting circuit depends on the interaction of two co-expressed lambdoid phage-derived proteins: the first is unstable and inhibits the regulatory activity of the second, stable protein. At the end of the pulse the unstable inhibitor protein disappears from the cell and the second protein triggers the recording of the event completion. Using stochastic simulation we showed that the proposed design can detect the completion of the pulse irrespective to the pulse duration. In our simulation we also showed that fusing the pulse detector with a phage lambda memory element we can construct a counter which can be extended to count larger numbers. The proposed design principle is a new control mechanism for synthetic biology which can be integrated in different circuits for identifying the completion of an event.
Journal Article
An Evolutionary Computational Approach to Humanoid Motion Planning
بواسطة
Hettiarachchi, Dhammika Suresh
,
Iba, Hitoshi
في
Asymmetry
,
Balancing
,
Biological evolution
2012
The theme of our work is centred on humanoid motion planning and balancing using evolutionary computational techniques. Evolutionary techniques, inspired by the Darwinian evolution of biological systems, make use of the concept of the iterative progress of a population of solutions with the aim of finding an optimally fit solution to a given problem. The problem we address here is that of asymmetric motion generation for humanoids, with the aim of automatically developing a series of motions to resemble certain predefined postures. An acceptable trajectory and stability is of the utmost concern in our work. In developing these motions, we are utilizing genetic algorithms coupled with heuristic knowledge of the problem domain. Unlike other types of robots, humanoids are complex in both construction and operation due to their myriad degrees of freedom and the difficulty of balancing on one or more limbs. The work presented in this paper includes the adopted methodology, experimental setup, results and an analysis of the outcome of a series of evolutionary experiments conducted for generating the said asymmetric motions.
Journal Article
Reverse engineering gene regulatory network from microarray data using linear time-variant model
بواسطة
Noman, Nasimul
,
Iba, Hitoshi
,
Kabir, Mitra
في
Cyclic AMP - metabolism
,
Dictyostelium - genetics
,
Dictyostelium - metabolism
2010
Gene regulatory network is an abstract mapping of gene regulations in living cells that can help to predict the system behavior of living organisms. Such prediction capability can potentially lead to the development of improved diagnostic tests and therapeutics. DNA microarrays, which measure the expression level of thousands of genes in parallel, constitute the numeric seed for the inference of gene regulatory networks. In this paper, we have proposed a new approach for inferring gene regulatory networks from time-series gene expression data using linear time-variant model. Here, Self-Adaptive Differential Evolution, a versatile and robust Evolutionary Algorithm, is used as the learning paradigm.
To assess the potency of the proposed work, a well known nonlinear synthetic network has been used. The reconstruction method has inferred this synthetic network topology and the associated regulatory parameters with high accuracy from both the noise-free and noisy time-series data. For validation purposes, the proposed approach is also applied to the simulated expression dataset of cAMP oscillations in Dictyostelium discoideum and has proved it's strength in finding the correct regulations. The strength of this work has also been verified by analyzing the real expression dataset of SOS DNA repair system in Escherichia coli and it has succeeded in finding more correct and reasonable regulations as compared to various existing works.
By the proposed approach, the gene interaction networks have been inferred in an efficient manner from both the synthetic, simulated cAMP oscillation expression data and real expression data. The computational time of this approach is also considerably smaller, which makes it to be more suitable for larger network reconstruction. Thus the proposed approach can serve as an initiate for the future researches regarding the associated area.
Journal Article
Evolutionary computation in gene network research
بواسطة
Iba, Hitoshi
,
Noman, Nasimul
في
Evolutionary computation
,
Genetic programming (Computer science)
2016
This book serves as a handbook for gene regulatory network research using evolutionary algorithms, with applications for computer scientists, biologists, and bioinformatics researchers This book compiles progress on gene regulatory network (GRN) research, focusing particularly on different domains that apply evolutionary algorithms (EAs) as the computational methodology. These areas are the analysis of gene expression data to discover knowledge; the reconstruction of GRN from expression profiles; and the evolution of GRN for target behavior. The book also presents uses of GRN with EAs in applications such as architectural design, agent control and robotics. The first part of the book introduces GRN to readers with a computer science background, and EAs to readers with a life science background. The authors present the EA approaches for analysis of gene expression data. Next, readers are guided step-by-step through the reverse engineering and evolution of GRN using EAs. Topics covered include deterministic and stochastic modelling of GRN, time series data analysis, single and multi-objective genetic algorithms, and swarm intelligence. The last part of the book focuses on future applications of GRN with use of EAs, in the fields of agent control, robotics, and design. The fifteen chapters are authored by well-known researchers and experienced practitioners in their respective fields. * Provides a reference for current and future research in gene regulatory networks (GRN) using evolutionary algorithms (EAs) * Covers all sub-domains of GRN research using EAs, such as expression profile analysis, reverse engineering, GRN evolution, applications * Contains useful contents for courses in gene regulatory networks, systems biology, computational biology, and synthetic biology * Delivers state-of-the-art research in genetic algorithms, genetic programming, and swarm intelligence Evolutionary Computation in Gene Network Research is a great resource for students, researchers, and professionals in computer science, systems biology, and bioinformatics. Hitoshi Iba is a Professor in the Department of Information and Communication Engineering, Graduate School of Information Science and Technology, at the University of Tokyo. He is an Associate Editor of the IEEE Transactions on Evolutionary Computation and the Journal of Genetic Programming and Evolvable Machines. Nasimul Noman is a lecturer in the School of Electrical Engineering and Computer Science at the University of Newcastle, NSW, Australia. From 2002 to 2012 he was a faculty member at the University of Dhaka, Bangladesh. He is an Editor of the BioMed Research International Journal. His research interests include computational biology, synthetic biology, and bioinformatics.
eBook
Evolving Robust Gene Regulatory Networks: e0116258
2015
Design and implementation of robust network modules is essential for construction of complex biological systems through hierarchical assembly of 'parts' and 'devices'. The robustness of gene regulatory networks (GRNs) is ascribed chiefly to the underlying topology. The automatic designing capability of GRN topology that can exhibit robust behavior can dramatically change the current practice in synthetic biology. A recent study shows that Darwinian evolution can gradually develop higher topological robustness. Subsequently, this work presents an evolutionary algorithm that simulates natural evolution in silico, for identifying network topologies that are robust to perturbations. We present a Monte Carlo based method for quantifying topological robustness and designed a fitness approximation approach for efficient calculation of topological robustness which is computationally very intensive. The proposed framework was verified using two classic GRN behaviors: oscillation and bistability, although the framework is generalized for evolving other types of responses. The algorithm identified robust GRN architectures which were verified using different analysis and comparison. Analysis of the results also shed light on the relationship among robustness, cooperativity and complexity. This study also shows that nature has already evolved very robust architectures for its crucial systems; hence simulation of this natural process can be very valuable for designing robust biological systems.
Journal Article
Evolving Architectures with Gradient Misalignment toward Low Adversarial Transferability
بواسطة
Pora, Wanchalerm
,
Iba, Hitoshi
,
Kera, Hiroshi
في
Artificial neural networks
,
Classifiers
,
Computer architecture
2021
Deep neural network image classifiers are known to be susceptible not only to adversarial examples created for them but even those created for others. This phenomenon poses a potential security risk in various black-box systems relying on image classifiers. The reason behind such transferability of adversarial examples is not yet fully understood and many studies have proposed training methods to obtain classifiers with low transferability. In this study, we address this problem from a novel perspective through investigating the contribution of the network architecture to transferability. Specifically, we propose an architecture searching framework that employs neuroevolution to evolve network architectures and the gradient misalignment loss to encourage networks to converge into dissimilar functions after training. Our experiments show that the proposed framework successfully discovers architectures that reduce transferability from four standard networks including ResNet and VGG, while maintaining a good accuracy on unperturbed images. In addition, the evolved networks trained with gradient misalignment exhibit significantly lower transferability compared to standard networks trained with gradient misalignment, which indicates that the network architecture plays an important role in reducing transferability. This study demonstrates that designing or exploring proper network architectures is a promising approach to tackle the transferability issue and train adversarially robust image classifiers.
Paper
A Brief Introduction to Evolutionary and other Nature‐Inspired Algorithms
بواسطة
Noman, Nasimul
,
Iba, Hitoshi
في
artificial intelligence
,
evolutionary computation
,
evolutionary programming
2016
This chapter presents an introduction of evolutionary and other nature‐inspired computation. The most prominent types of evolutionary computation (EC) are genetic algorithms (GA), genetic programming (GP), evolutionary strategy (ES) and evolutionary programming (EP). Many computational algorithms and problem‐solving techniques, commonly known as swarm intelligence, have been developed by simulating the coordination and teamwork strategies in social insects. Elements comprising GAs are data representation, selection, crossover, mutation, and alternation of generations. GP can be used to apply evolutionary approaches to automatic code generation and problem solving by artificial intelligence. ES in its early days differed from GAs in the following two ways: mutation is used as the main operator and real number expressions are handled. The chapter also discusses relative advantages/disadvantages and application areas of these algorithms. Numerous applications of EC exist in structural engineering, architectural design, environmental engineering, geotechnical and water resource engineering.
Book Chapter